Reconstructing Signing Avatars From Video
Using Linguistic Priors
Abstract
Sign language (SL) is the primary method of communication for the 70 million Deaf people around the world. Video dictionaries of isolated signs are a core SL learning tool. Replacing these with 3D avatars can aid learning and enable AR/VR applications, improving access to technology and online media. However, little work has attempted to estimate expressive 3D avatars from SL video. This task is difficult due to occlusion, noise, and motion blur. We address this by introducing novel linguistic priors that are universally applicable to SL and provide constraints on 3D hand pose that help resolve ambiguities within isolated signs. Our method, SGNify, captures fine-grained hand pose, facial expression, and body movement fully automatically from in-the-wild monocular SL videos. We evaluate SGNify quantitatively by using a commercial motion-capture system to compute 3D avatars synchronized with monocular video. SGNify outperforms state-of-the-art 3D body-pose- and shape-estimation methods on SL videos. A perceptual study shows that SGNify’s 3D reconstructions are significantly more comprehensible and natural than those of previous methods and are on par with the source videos.
Video
Poster
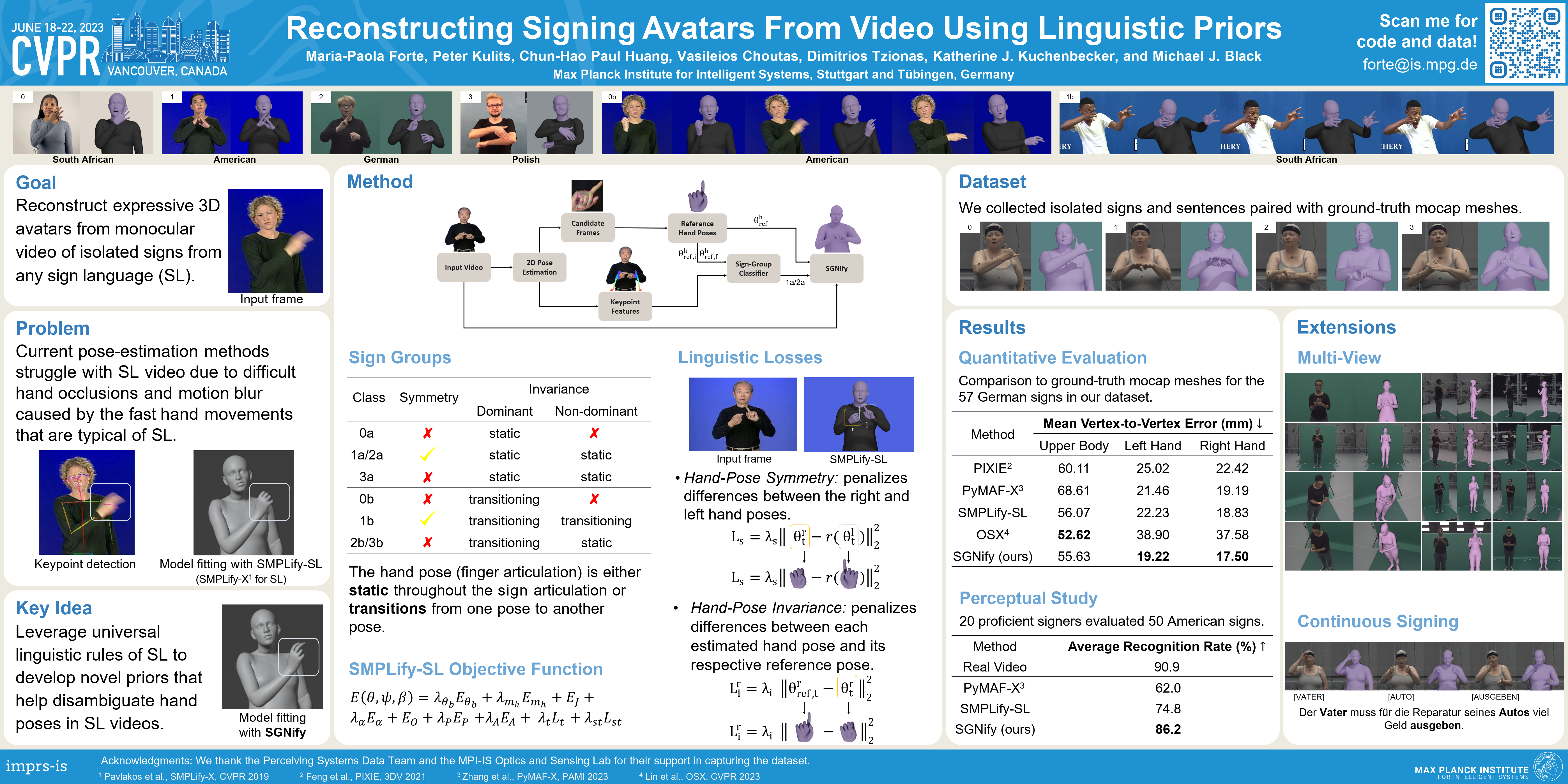
BibTex
@inproceedings{Forte23-CVPR-SGNify, title = {Reconstructing Signing Avatars from Video Using Linguistic Priors}, author = {Forte, Maria-Paola and Kulits, Peter and Huang, Chun-Hao Paul and Choutas, Vasileios and Tzionas, Dimitrios and Kuchenbecker, Katherine J. and Black, Michael J.}, booktitle = {IEEE/CVF Conf.~on Computer Vision and Pattern Recognition (CVPR)}, month = jun, year = {2023}, pages = {12791-12801}
}
Contact
For questions, please contact forte@is.mpg.de